<--Back to Wiki Home
Define Statistical Regression Analysis in Real Estate
Statistical Regression Analysis:
"Statistical regression analysis" is a method used to find a relationship between different pieces of information, like how one thing might affect another. It helps us make predictions or understand how things are connected by looking at patterns in data. By studying these patterns, we can estimate how changes in one thing might lead to changes in another.
Example:
A real estate appraiser is trying to figure out what affects the price of houses in a neighborhood. They collect data on house prices, square footage, the number of bedrooms, and the age of the houses. Using statistical regression analysis, the appraiser can identify the relationship between these factors and the house prices. They might find that larger houses with more bedrooms tend to have higher prices, while older houses might have lower prices. This information can help them better estimate the value of a house based on its specific features.
"A Deep Dive for Real Estate Appraisers"
Here's more in-depth information about statistical regression analysis and its relevance to real estate appraisal:
Types of Regression: There are various types of regression analysis, but the most common one used in real estate appraisal is linear regression. Linear regression helps identify a straight-line relationship between an independent variable (e.g., square footage) and a dependent variable (e.g., house price). Other types include multiple regression (involving multiple independent variables) and nonlinear regression (for more complex relationships).
Coefficient of Determination (R-squared): R-squared is a statistical measure that indicates how well the regression line fits the data. It ranges from 0 to 1, with values closer to 1 indicating a better fit. A high R-squared value means the model can explain a large portion of the variation in the dependent variable, making it more reliable for predictions.
Assumptions: Regression analysis relies on certain assumptions, such as linearity (a straight-line relationship between variables), normal distribution of errors, and independence of observations. Violating these assumptions can lead to inaccurate or misleading results.
Limitations: Statistical regression analysis is not foolproof. It can be sensitive to outliers (data points that are very different from the rest), and it may not capture all factors influencing the dependent variable. It's crucial to use the method with caution and consider other factors and expert judgment in the appraisal process.
Application: Real estate appraisers use statistical regression analysis to create valuation models that estimate property values based on various factors. These models help them analyze market trends, make predictions, and provide more accurate property appraisals.
Understanding statistical regression analysis is essential for real estate appraisers because it allows them to create data-driven models for estimating property values. However, it's crucial to remember that it's only one tool in the appraisal process, and professional judgment, experience, and knowledge of the local market are still vital components of an accurate appraisal.
Let's consider a real-world example of an appraiser using statistical regression analysis:
An appraiser, Jane, is tasked with estimating the value of a three-bedroom, 2,000 square-foot house in a suburban neighborhood. To determine the value accurately, she needs to consider factors that impact the price of similar properties in the area.
Jane collects data on recent home sales in the neighborhood, including the sale prices, square footage, number of bedrooms, number of bathrooms, age of the house, and proximity to schools. She then uses statistical regression analysis to understand the relationship between these factors and the sale prices of the properties.
After analyzing the data, Jane finds the following insights:
The price of a house increases by $100 per square foot.
An additional bedroom adds $20,000 to the house's value.
An additional bathroom adds $15,000 to the house's value.
Every year of age decreases the house's value by $1,000.
Proximity to a well-ranked school adds $10,000 to the house's value.
With these insights, Jane can now estimate the value of the three-bedroom, 2,000 square-foot house. Using the regression analysis results, she calculates the estimated value as:
(2,000 sq.ft. * $100) + (3 bedrooms * $20,000) + (2 bathrooms * $15,000) - (10 years * $1,000) + $10,000 (for the well-ranked school) = $200,000 + $60,000 + $30,000 - $10,000 + $10,000 = $290,000
Jane concludes that the estimated value of the house is $290,000 based on her statistical regression analysis. However, she still considers other factors, such as current market trends and any unique features of the property, to ensure an accurate appraisal.
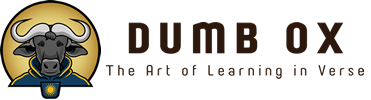
"Wit & Whimsy with the Dumb Ox: Unlocking Knowledge with Rhyme:"
In a world of numbers and facts,
Regression helps us see the impacts.
It shows us how things relate,
For appraisers, it's truly great.
House size, age, and rooms galore,
Price connections we can't ignore.
A pattern's found, the story's clear,
Regression guides us, never fear.
So when you seek a value to assign,
Statistical regression can help you find,
The hidden links that weave and bind,
A useful tool, for the appraiser's mind.